The artificial intelligence model predicts patients’ heart disorders and risk of future heart failure using just an electrocardiogram.
Edis Mesic
Staff Reporter
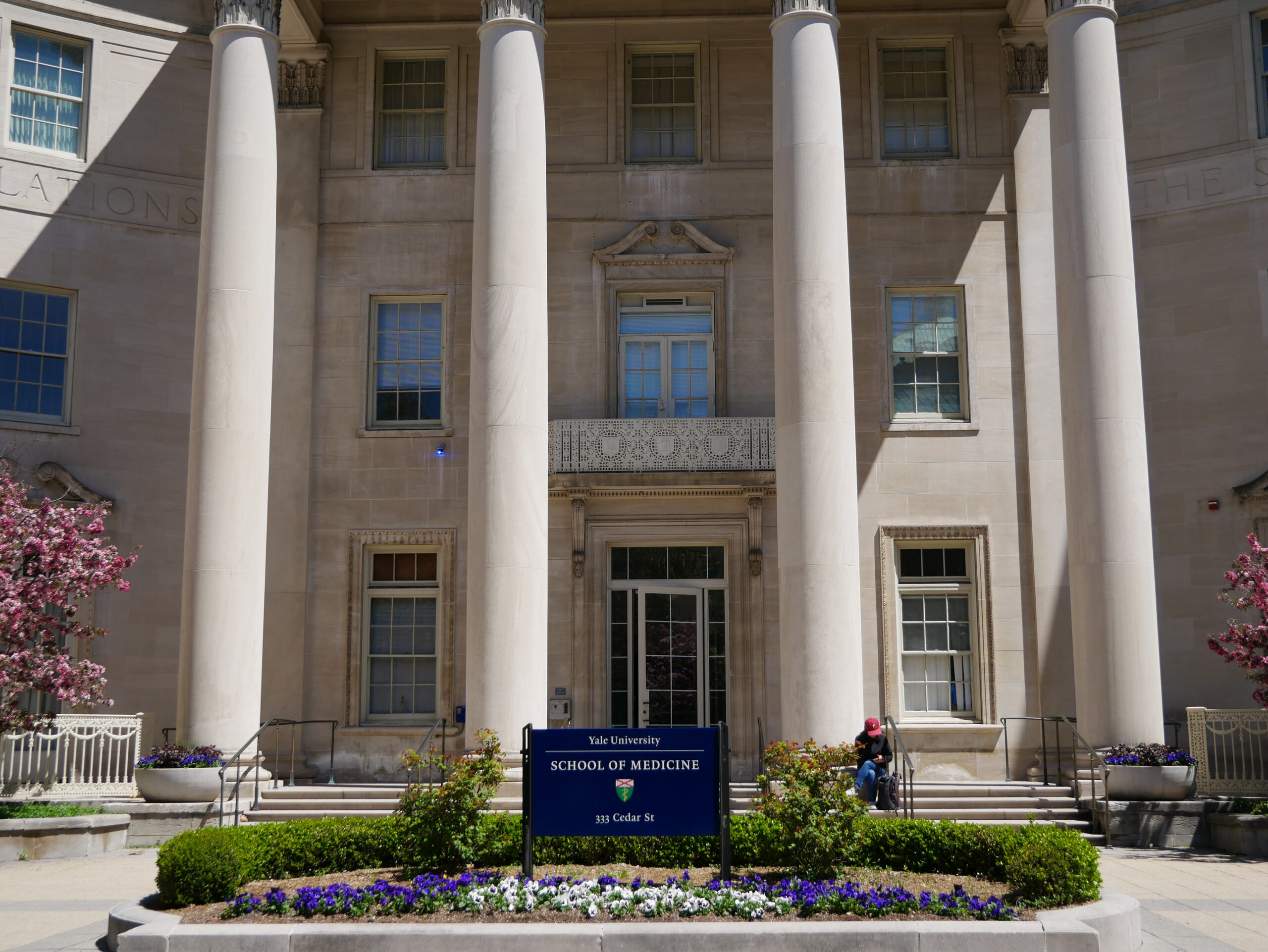
YuLin Zhen, Photography Editor
A team of Yale researchers at the Cardiovascular Data Science Lab at the School of Medicine recently published a study showcasing a new artificial intelligence tool for predicting the risk of heart failure.
The team’s research initially focused on a computer vision model that processed the visual data from an electrocardiogram, or ECG, and detected the presence of left ventricular systolic dysfunction or LVSD, a heart disorder typically not visible on any ECG.
“I always wanted to develop tools that increase the accessibility of diagnostics across the world, which is why the focus on ECG images,” noted Dr. Lovedeep Singh Dhingra, the first author of the study and a postdoctoral fellow at the CarDS Lab. “A lot of research in the last few years has shown that ECGs are a richer tool than we give them credit for.”
The only other way of screening patients for this condition is through a transthoracic echocardiogram, an ultrasound of the heart that captures any abnormalities in its structure or function.
The imaging technique is not widely available and requires special expertise to read.
Through an AI tool that trained the model to pick up on previously undetectable signals on a scannable ECG, the team became the first to find a mechanism to pick up LVSD from an ECG image alone and published their first study in 2023. The breakthrough allowed anyone with a smartphone to simply take a photo of an ECG and put it into the model to predict low ejection fraction, a key marker of LVSD.
The team’s next step was to determine if the model for detecting current heart failure in patients could detect signals that predicted future heart failure risk as well. Dr. Arya Aminorroaya, co-author of the study, noted that while risk stratification models that assess heart failure risk do exist, they require a multitude of markers that are difficult to obtain.
“If we can predict the risk of heart failure using this simple ECG, then we could transform the way that we risk stratify patients for heart failure, and we may be able to start patients on therapies sooner rather than later,” Aminorroaya said.
In their research, the team discovered an interesting phenomenon. Patients who tested false positives with the AI model — meaning that the model detected low ejection fraction when the echocardiogram did not — tended to develop heart failure in the years that followed more often than patients who screened negative.
This discovery proved the team’s hypothesis that the AI tool was capturing a signal that electrocardiograms could not confirm but that was successfully predicting the risk of heart failure.
After this milestone, the researchers continued their work by testing their model in both clinical and population settings.
The team used data from more than 200,000 patients at Yale New Haven Hospital but frequently ran into the issue of patients switching between hospitals and creating gaps in the long-term clinical data. On the other hand, the team collected data from 40,000 patients at UK Biobank and 13,000 patients from ELSA-Brazil who were systematically followed up for heart failure.
“We had different types of data adjudication across different sources, and the model performed consistently across those different definitions, which is why the model did even better compared to a typical score that would be used for heart failure prediction,” Dhingra said.
The working model is currently hosted on the CarDS lab website, where people can freely use it for research purposes.
Looking to the future, Dhingra sees the project going in three possible directions.
The first is targeting other heart diseases like valve disorders or hypertrophy through a model that functions as a single, broad screen for structural heart disease. The second is not just inputting clinical ECGs into their model but also testing variable ECGs produced by wearable portable devices like an Apple Watch, allowing people without established health care to screen for future heart failure. The third is running randomized trials to test the clinical effectiveness of the prediction model.
“The work of the CarDS Lab is focused on using AI to specifically change the landscape of care in low-resource settings where both equipment and expertise are limited,” Dr. Rohan Khera, senior author of the study and director of the CarDS Lab, wrote to the News.
To learn more about ongoing research at the CarDS lab, see here.