Healthcare and Artificial Intelligence (AI) synergise well, but aligning these two domains presents several daunting challenges. AI systems require massive amounts of high-quality data to achieve meaningful healthcare outcomes, but integrating data from multiple medical sources while addressing ethical and practical considerations is difficult.
Anurag Patil is a respected machine-learning, software engineering, and biomedical research expert leading efforts to bridge the gap between these disciplines. A trailblazer in AI-driven healthcare solutions, Patil’s valuable contributions to AI applications and data engine creation are helping transform the healthcare landscape.
Perhaps most significantly, Patil’s work inspires new levels of cross-discipline collaboration. This means that some of the greatest minds in healthcare are working together to help solve patient issues.
Collaboration is the key to success in this all-important industry—and Patil is leading the way.
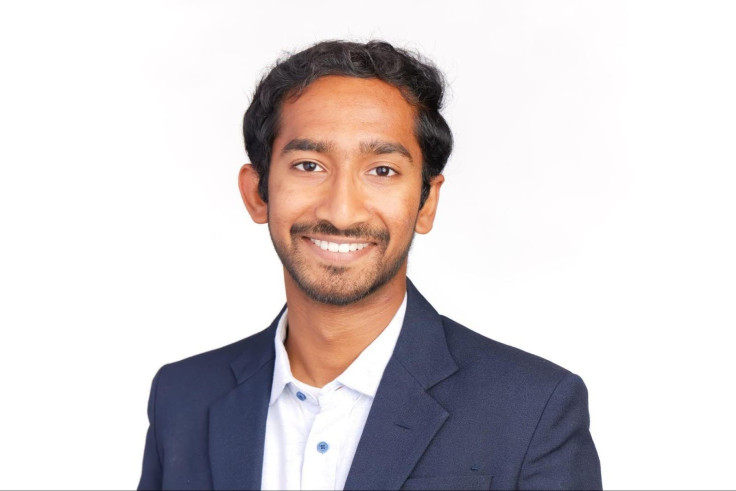
The Complexity of AI in Healthcare
From predicting disease outbreaks to discovering biomarkers in real-time, AI has demonstrated its potential to address critical healthcare challenges.
However, several legitimate hurdles remain to widespread adoption:
- Much of modern medical data is unstructured and fragmented across systems
- Some of the data remains inaccessible for analysis
- Machine-learning models require repeated fine-tuning to address the many nuances of biomedical contexts
This final point demands significant technical expertise and domain-specific knowledge. “The true challenge isn’t just creating machine-learning models—it’s building a system where these models can extract actionable insights from complex, unstructured clinical data,” Patil explains.
Patil recognised these challenges early on and has focused on them throughout his career. His top priority is bridging the expansive gap between engineering and biomedical research to craft solutions that carefully maximise AI’s potential in healthcare.
Anurag Patil: Blending Engineering and Medicine
Patil’s commitment to solving healthcare’s most challenging problems is equalled only by his love of learning. He has a Master’s in Computational Science, Engineering, and Mathematics from UT Austin, where he specialises in applying deep learning to biological systems.
After graduating, Patil worked at a major American electric vehicle manufacturer in Palo Alto, California, where he focused on optimising data ingestion systems. This role taught him how to process and analyse large-scale datasets.
Currently serving as a Senior Data Engineer at a primary healthcare system in New York City, Patil oversees the development of sturdy data pipelines and fine-tunes machine-learning models for the hospital network. His work now transcends conventional data integration.
Patil focuses on creating tools for scalable and highly efficient AI solutions for healthcare: “I engineered a large-scale platform for health data, including Electronic Health Records (EHR), pathology, and radiology data.”
This accomplishment shows Patil’s strong leadership in building streamlined systems for diverse medical datasets.
Data Engine Creation: The Backbone of AI in Healthcare
Data engine creation is a critical yet often overlooked component of healthcare AI, and Patil has designed systems that aggregate, process, and standardise medical data. This leads to advanced AI applications that detect diseases early on and plan personalised patient treatments.
One of Patil’s standout projects is the “datapilot” module. This groundbreaking module automates processing several distinct datasets, including those from EHRs, radiology, pathology, and even electroencephalogram (EEG) data. This valuable innovation enhances data accessibility for research teams so they can gain faster insights into complex medical challenges.
‘Our goal is to create systems where researchers can access and analyse data in real-time, without the bottlenecks of traditional data processing,’ says Patil.
A Case Study in Innovation: Biomarker Discovery
Patil’s work on biomarker discovery has been truly transformative. At the leading healthcare system, he has fine-tuned two Large Language Models (LLMs) used to diagnose and monitor diseases, GPT-4 and LLaMA 3.1. ‘Typically, clinicians spend 15–30 minutes reviewing each case for biomarkers,’ Patil explains. ‘With the trained LLMs and the custom framework we developed, this process now takes seconds.’
Notably, the LLaMA model demonstrated cost-effectiveness by matching GPT -4’s performance when fine-tuned with the appropriate dataset. This critical integration has since allowed research teams to gain increasingly rapid and accurate insights. As a result, healthcare studies have become less time-consuming and more substantial.
The Importance of Interdisciplinary Collaboration
Patil’s secret to success is his ability to seamlessly integrate across engineering, data science, and biomedical research. He starts by understanding the specific needs of healthcare professionals before aligning those needs with specific technical solutions. It sounds simple, especially for such a complex field, but his approach has allowed Patil to overcome several obstacles.
‘Interdisciplinary collaboration is essential for creating practical AI tools in healthcare. It’s not just about training models—it’s about ensuring they deliver actionable, real-world results,’ says Patil.
His knack for navigating these complexities shows how vital collaboration is in advancing healthcare technology.
Shaping the Future of AI in Healthcare
As he looks to the future of AI in healthcare, Anurag Patil knows the best is yet to come.
Developing ethical and interpretable AI systems will be challenging, but it’s possible. He wants to help build tools that increase transparency in machine-learning models so healthcare professionals will trust and better comprehend AI-generated insights more confidently.
Beyond technical advancements, Patil hopes to lead several top-tier collaborative projects. He believes these endeavours will shape the generation of AI-driven healthcare tools: ‘The future of healthcare depends on our ability to harness AI responsibly and effectively. My goal is to contribute solutions that not only solve technical challenges but also address the broader ethical considerations of AI in medicine.’
As AI continues transforming medicine, Anurag Patil’s contributions showcase the potential of interdisciplinary collaboration and have ushered in the next wave of AI-driven solutions in healthcare. They’re precise, efficient, cost-effective, and, most importantly, they continue to improve patients’ lives worldwide.